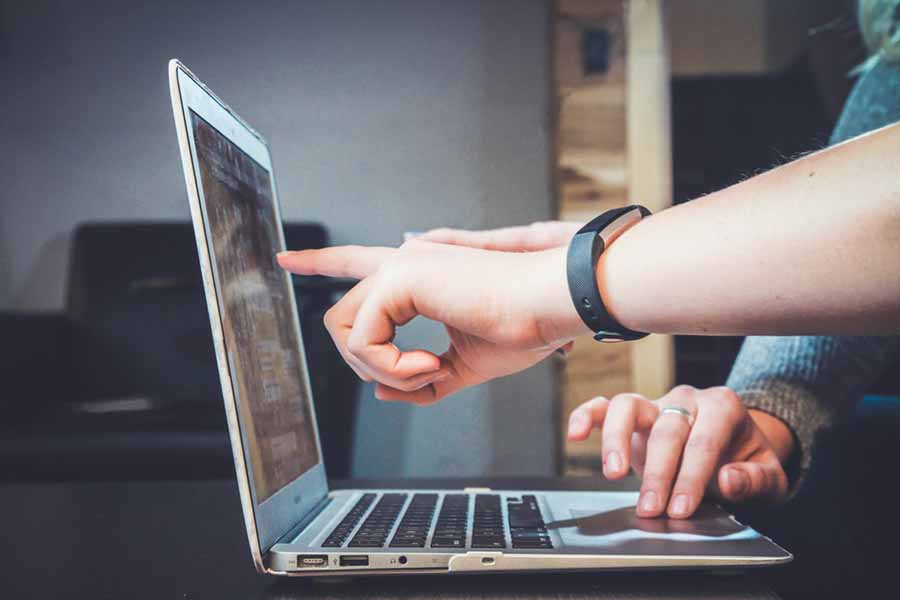
guidance on ai in manufacturing
The recent progress in artificial intelligence (AI) and machine learning (ML) has brought both a lot of attention and a lot of concern to this emerging field of technology and its applications. While the potential of AI has been debated from various perspectives at international forums as well as in the public media, this has also brought some spectacular speculations of the future perspectives. Specific focus here is on complications for society if the full potential of AI is released without oversight and guards. While this debate is ongoing and will very likely continue for some time, it is also clear that AI has a very high potential to serve us. The facilitation of tasks and provision of decision support is a key driver in the manufacturing industry.
As with all new technologies, the adoption of AI and ML for industrial manufacturing will depend on the development of standards. This will include both standards that specify requirements and establish best practices for the functionality of AI and ML systems, and also standards that enable AI and ML systems to operate as an integrated part of the industrial IT systems. This need for several layers of standards has brought initiatives for mapping existing relevant standards, road mapping the developing needs, and coordinating the development of new AI and ML standards, to be launched at several organizations worldwide. At present, while AI and ML are by comparison a fairly new topic for standardization, the absolute majority of the standards that have been identified as relevant for this topic are of the type that are needed to enable AI and ML to be integrated as a part of the industrial IT systems. However, since the scope of AIRISE is to support European SMEs to adopt AI for efficient use in production, we will focus on standards and standardization activities that are most relevant to support practical use od AI by SMEs in the European market.
selected standards on ai in manufacturing
CEN/CLC/JTC 21 - published standards
We have selected a few standards for you to orienteer yourself:
- CEN/CLC ISO/IEC/TR 24027:2023 Information technology - Artificial intelligence (AI) - Bias in AI systems and AI aided decision making: This technical Report (TR) was originally developed and published by ISO/IEC JTC 1/SC 42 in 2020 and has been adopted by CEN/CLC/JTC 21 as a European standardization document, making it a mandatory Technical Report for reference and publication by all member countries. This document addresses bias in relation to AI systems, especially with regards to AI-aided decision-making. Measurement techniques and methods for assessing bias are described, with the aim to address and treat bias-related vulnerabilities. All AI system lifecycle phases are in scope, including but not limited to data collection, training, continual learning, design, testing, evaluation and use.
- CEN/CLC ISO/IEC/TR 24029-1:2023 Artificial Intelligence (AI) - Assessment of the robustness of neural networks - Part 1: Overview: This technical Report (TR) was originally developed and published by ISO/IEC JTC 1/SC 42 in 2021 and has been adopted by CEN/CLC/JTC 21 as a European standardization document, making it a mandatory Technical Report for reference and publication by all member countries. This document provides background about existing methods to assess the robustness of neural networks.
- EN ISO/IEC 22989:2023 Information technology - Artificial intelligence - Artificial intelligence concepts and terminology: Originally developed and published by ISO/IEC JTC 1/SC 42 in 2022 and has been adopted by CEN/CLC/JTC 21 as a European standard, making it a mandatory national standard for all member countries. This document establishes terminology for AI and describes concepts in the field of AI. This document can be used in the development of other standards and in support of communications among diverse, interested parties or stakeholders. This document is applicable to all types of organizations (e.g. commercial enterprises, government agencies, not-for-profit organizations).
- EN ISO/IEC 23053:2023 Framework for Artificial Intelligence (AI) Systems Using Machine Learning (ML): Originally developed and published by ISO/IEC JTC 1/SC 42 in 2022 and has been adopted by CEN/CLC/JTC 21 as a European standard, making it a mandatory national standard for all member countries. This document establishes an Artificial Intelligence (AI) and Machine Learning (ML) framework for describing a generic AI system using ML technology. The framework describes the system components and their functions in the AI ecosystem. This document is applicable to all types and sizes of organizations, including public and private companies, government entities, and not-for-profit organizations, that are implementing or using AI systems.
- EN ISO/IEC 23894:2024 Information technology - Artificial intelligence - Guidance on risk management: Originally developed and published by ISO/IEC JTC 1/SC 42 in 2023 and has been adopted by CEN/CLC/JTC 21 as a European standard, making it a mandatory national standard for all member countries. This document provides guidance on how organizations that develop, produce, deploy or use products, systems and services that utilize artificial intelligence (AI) can manage risk specifically related to AI. The guidance also aims to assist organizations to integrate risk management into their AI-related activities and functions. It moreover describes processes for the effective implementation and integration of AI risk management. The application of these guidance can be customized to any organization and its context.