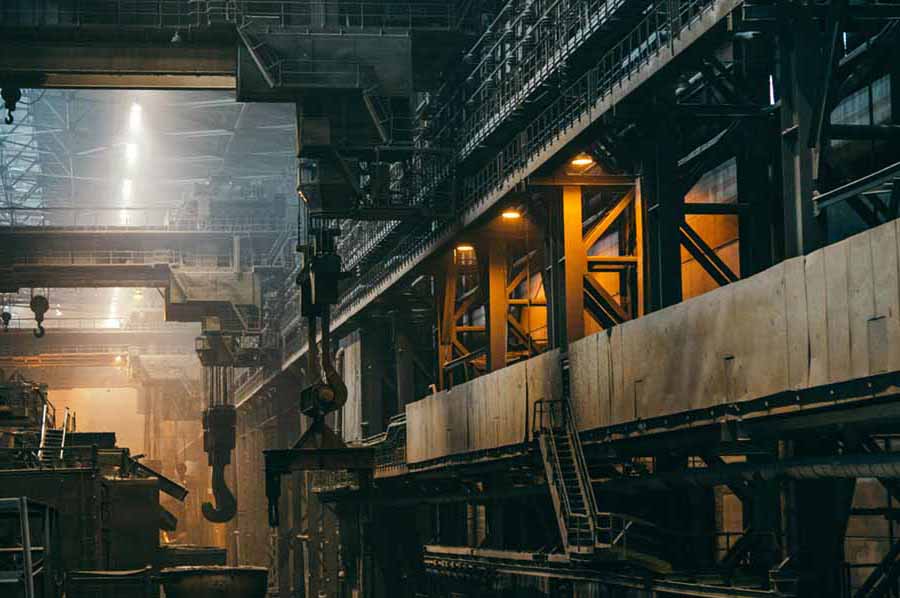
Industrial use or Artificial Intelligence
stories about AI-in-manufacturing
Artificial Ingelligence (AI) has created quite a few advacements in manufacturing, some of them well known to the public, others being exploited more silently. We have collected some of these stories to flag the diversity and to show the opportunities of using AI in manufacturing.
Find inspiration for your organisation and your own shop-floor. For next steps, just drop us a line.
design manufacturing operations monitoring and control production chain supply chain
GM and Autodesk use AI for Biometric Design of Structural Components
a story on using AI in design
Engineering design workflows are nowadays well established; the whole process consists of multiple steps and requires both product, material and process knowledge, as well as experience and expertise. As such, engineering design is one of the most resource intensive tasks for a manufacturing company. Typically engineering design comprises different steps:
- Conceptual design
- Proof of concept
- Design refinement
- CAE validation
- Manufacturing/production sign off
For each step of the aforementioned process, extensive technical knowledge is needed. Even if sophisticated software is employed, in order to produce a useful design that is prepared for manufacturing, comprehensive, domain-specific knowledge must be utilized to precisely describe each dimension, specification, and feature. In addition, feedback loops between design, manufacturing and validation phases introduce complexity and delays, typically linked to resource waste and slower time to market a new product.
AI-enabled tools can offer an appealing solution, accelerating engineering design and potentially saving valuable resources. A particularly interesting field is Generative Design, that allows engineers to explore a larger, more diverse design space for a given problem. Generative Design tools use algorithms to generate a number of different design variants within the limitations imposed by the operator. The design problem is formulated as a function of design criteria, while certain optimization objectives are also set (for example maximize stiffness-to-weight ratio). The software tool creates multiple design variants, from which the operator can select the ones that better fit their purpose; be it manufacturing process limitations or other preferences. AI can assist this process by assessing the viability of the generated solutions and proposing only the more appropriate ones to the operator. Based on operator responses, the AI-enabled system can further tailor the selection criteria so that an optimal design will emerge at the end.
Characteristic examples include biomimetic designs for structural components; GM and Autodesk have used such approaches to design a structurally optimal seat bracket (among others). Generative design was deployed to generate 150 design alternatives, coupled with AI to select the optimal. Similar approaches have been followed in design optimization of heat exchange interfaces/devices, where the resulting complex geometries generated by computer software could not be conceived by a human designer. This approach can be extended beyond a single part (or product) design, to designing layouts for manufacturing lines, infrastructure as well as facilities like power generation and distribution grids.
Such tools are not expected to completely remove humans from the design loop; however, they can simplify, automate and reduce the time and effort required for initial design iterations that create bottlenecks.
Curious about what AI can do for you in design?
Reduction of Labour Cost through the Use of AI in Digitised Workshops
a story on using AI to increase productivity
Manufacturing operations entail every manufacturing process required to produce goods for consumers with a goal of optimizing efficiency. Some of these processes include:
- Quality Management
- Manufacturing Execution Systems
- R&D Management
- Advanced Inventory Planning & Scheduling
- Facility & Machine Maintenance
For decades, factories have been “digitizing” their production lines with distributed and oversight systems. Maintaining appropriate processes throughout a factory's manufacturing operations is crucial for meeting customer demand and ensuring the success of a business. The benefits of fitting manufacturing operations for a factory include a higher level of safety & quality control, ability to detect issues quickly, reduction of waste and simplification of the production operation.
A change in market supply or market demand can occur on short notice, so there’s a need for just-in-time manufacturing processes aided by insights from suitable manufacturing operation management software. These operational functionalities must be integrated into the shopfloor seamlessly through process automation. Therefore, a promising way of optimizing the workflow of manufacturing operations is to implement AI technologies in the digital management tools.
One great example can be at factory level, where operators rely on their knowledge and intuition to monitor a multitude of signals across various machines to operate the equipment. This means that troubleshooting, testing, and other tasks fall on the operators as well, which adds to their workload. This can lead to problems like human error leading to reduction of overall manufacturing efficiency but also on big reliance on experienced workers, which makes it difficult to replace them once they go on retirement.
With AI, manufacturers can reduce labor costs significantly while improving overall productivity and efficiency at their factories. Other applications include:
- Automate complex and repetitive tasks at factory level
- Reduce cycle times Improve workplace safety with oversight systems
- Quick detection and notification of shopfloor problems
- Reduce the experience factor required to operate a factory
- React quickly to demand fluctuations with alert systems
Curious what AI can do for you on best-in-class manufacturing operations?
AI-based Problem Finding in a Welding Workshop
a story on using data from the manufacturing environment
Monitoring at shop floor level is mostly done to ensure smooth operation of manufacturing processes. Product quality and production time are the key objectives as any deviation from the plan creates unforeseen cost. In most cases, such monitoring is used to trigger repair of machines or inspection for produced products. Advanced uses of monitoring results however allow planning of preventative maintenance or in-situ rework of parts. As such, monitoring includes
- Acquisition of data relevant to manufacturing quality
- Identification of causes for product quality deviation
- Ability to computationally analyse data
- Visualise the result or automatically trigger control
Relevant data is a key term for this topic. Acquired data needs to describe what happens in the real world with sufficient detail in time and precision. Data rates need to be well aligned to process durations so that the relevant details of the process can be tracked. If additional data from the environment is acquired – like the environment temperature – then measurement intervals might be completely different. Still – especially when using AI – the impact of changes in the environment of the manufacturing process might not be known a priori.
An example for this is a welding process which produces defects in 10% of the produced parts. All known parameters were constant looking at the welding equipment, gas supply, raw material and process parameters. Monitoring the environment temperature and labelling the produced parts revealed that the process deviations happened at certain times of the day in correlation with a temperature drop or rise by 2-3 degrees centigrade, in some cases even 10 degrees. Finally, the cause for these deviations was found no to be the temperature of the environment itself, but an air stream caused by an opened door during delivery of goods. Here, there was no causal link between the environment temperature and the defects, but a causal link between the door sensor and the defect, all of which are at different time scales, different locations and even in different technical context.
This example shows that much data from diverse sources is needed to identify causal relations that are not known a priori. If AI is used to analyse the raw data, a huge potential arises that we as operators and entrepreneurs learn, that we discover and invent new solutions.
Curious about what AI can do for you in finding causes of quality issues?
AI-based Alternatives Planning for Instant Re-Scheduling and Robust Production
a story on using AI to create options before things happen
Production chain encompasses anything that links individual processes to each other; the term includes (but is not limited to) production planning and manufacturing execution systems. Such systems have become common place in manufacturing firms over the last 20 years, typically being the starting point of the application of digital solutions on the shop floor. While digitalization is the first step, existing systems are typically deterministic, applying a fixed logic and using historical averaged data, while relying upon user expertise.
AI can further enhance such tools. AI can be used to not only match product requirements with process/machines/workstations capabilities, but also to generate multiple alternative process plans and select the optimal trade off between contrasting priorities. In addition, due to AI being able to generate and select between multiple alternatives in very short time, it can be used for real-time re-scheduling based on data coming from the shopfloor, thus reacting rapidly to unexpected system behavior (delays or breakdowns in certain workstations). AI can also be utilized towards balancing or manufacturing resources, as well as towards energy-related planning and scheduling (avoiding peaks, prioritizing a greener energy mix).
The aforementioned approaches focus solely on time and energy; however, AI can also be used towards addressing quality-related metrics. For example, certain workstations may produce products of higher quality that need to be prioritized for certain uses/customers; data mining combined with AI can be deployed to detect such behaviors. In addition, AI can be used to monitor a certain property towards appropriate tolerance management and prevention of defect propagation along the production chain. AI can also be used to guide the cause analysis of such occurrences.
Such solutions can contribute to identification of production chain deficiencies, higher utilization rate of manufacturing resources, increased energy efficiency, increased product quality and reduced waste.
BMW Group utilizes image recognition software for production and quality evaluation, where the AI application determines deviations from the standard in real time and checks. As a result, they’ve achieved high levels of precision in manufacturing. Another company that’s benefited from AI in manufacturing is Porsche. They have deployed autonomous guided vehicles (AGVs) in their production line shopfloor to automate significant portions of automotive manufacturing.
If you want to discuss your AI-in-manufacturing application idea, then check out our services.
Curious about what AI can do for you on continuous production?
Amazon's AI-driven Shop-floor Logistics for Continuous Manufacturing Operations
a story on using AI for an adaptive material and part supply
The transfer of products and services from suppliers to customers must be planned, coordinated, and under strict supervision. This is known as supply chain management, and it is an essential component of corporate operations. Modern-day supply chains can be vastly complex, requiring information and material flows to be synchronized across the globe. In addition, volatility and market disruptions are commonplace, with manufacturing companies being left to deal with very complex problems. AI approaches can provide significant benefits to supply chain challenges as they are very capable in managing complexity.
Applications of AI-enabled tools focus around 3 main areas; Demand Forecasting, Routing Efficiency and Warehouse Space Utilization.
One of the key areas where AI can improve supply chain aspects is demand forecasting. AI can help develop diverse failure scenarios, as well as multiple variants for impact mitigation, making supply chains more resilient and aiding rapid and intelligent decision making should such scenarios materialize. AI can also be used to monitor indicators that could be used as early warnings for such scenarios and thus improve resilience.
AI-based inventory optimization can give warehouse managers insights on inventory levels and generate stocking recommendations utilizing data from production orders, purchase orders, and supplier deliveries, resulting in just-in-time restocking and avoiding both running out of materials or products as well as overstocking.
When linked together, both aforementioned areas can be used to mitigate the “bullwhip effect” (the effect of amplification of fluctuations in supply and demand as they move downstream the supply chain)
Another green field for AI is routing efficiency, significantly impacting both time as well as energy usage. With respect to internal/shopfloor logistics, multiple pieces of equipment (AGVs, forklifts) as well as humans are typically operating in the same space and during the same time, with a need to be synchronized to improve both efficiency and safety. AI combined with swarm navigation approaches can be deployed to provide individualized guidance for each part of the complete system. Such approaches can also be expanded to external logistics, by taking into account historical data, real-time traffic updates, weather conditions etc. to optimize routing and scheduling of deliveries.
Last but not least, AI approaches can be deployed for warehouse space utilization, augmenting existing software with AI based variant analysis towards an efficient warehouse management service.
Such solutions can contribute to increased supply chain resilience, faster reaction to external volatility, and more efficient internal and external logistics operations in terms of time and energy usage.
In order for logistics in a supply chain to be handled efficiently through AI & automation, companies like Amazon, Tusimple, and Nuro are extensively investing in transport automation technologies like autonomous trucks or cobots which are helping improve efficiency, productivity, and safety through automated warehouse management.
Curious about what AI can do for you about optimising the supply chain?